Artificial Intelligence and Twitter Could Be Used to Detect Early Signs of Mental Disorders
The findings are published in the journal Language Resources and Evaluation.
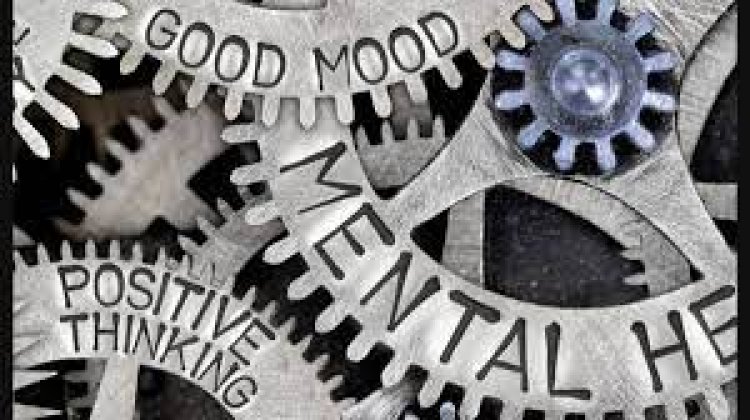
Researchers are in the process of developing anxiety and depression prediction models using artificial intelligence (AI) and Twitter, one of the world's largest social media platforms, that could detect symptoms of these conditions prior to clinical diagnosis.
Researchers at the University of So Paulo (USP) in Brazil reported that the model's preliminary results indicated the possibility of predicting a person's likelihood of developing melancholy based solely on their social media friends and followers.
The results are published in Language Resources and Evaluation journal.While there are numerous natural language processing (NLP) studies on melancholy, anxiety, and bipolar disorder, the majority of these studies analyzed English texts and did not match the profiles of Brazilians, according to the researchers.
In the first stage of this research, a database containing information about 47 million publicly posted Portuguese texts and the network of connections between 3,900 Twitter users was created and named SetembroBR. Before the survey, these users had reportedly been diagnosed with or treated for mental health issues. During the COVID-19 pandemic, these tweets were collected.
"Initially, we painstakingly collected timelines by analyzing the tweets of approximately 19,000 users, the population of a village or small town.
Then, we used two datasets, one for users who reported being diagnosed with a mental health issue and the other as a control sample. Professor at USP Ivandre Paraboni, the article's last author, stated, "We wanted to differentiate between depressed individuals and the general population."
The research also collected tweets from friends and followers because people with mental health issues tend to follow certain accounts, such as discussion forums, influencers, and celebrities who publicly acknowledge their depression.
The second stage, which is still in progress, has yielded some preliminary results, such as the possibility of determining a person's likelihood of developing depression based solely on their social media friends and followers, without considering their own posts.
The researchers used deep learning (AI) to create four text classifiers and word embeddings (context-dependent mathematical representations of the relationships between words) using models based on bidirectional encoder representations from transformers (BERT), a machine learning algorithm used for NLP.
These models resemble a neural network that learns contexts and meanings by observing sequential data relationships, such as the relationships between words in a sentence. The training input consisted of 200 randomly selected tweets from each user.
The researchers discovered that BERT performed the best among the models for predicting depression and anxiety. Due to the fact that the models analyzed sequences of words and complete sentences, it was possible to observe, for instance, that people with depression tended to write about subjects related to themselves, using first-person pronouns and phrases, as well as death, crisis, and psychology.
"The signs of depression that can be identified during a doctor's visit are not necessarily the same as those that appear on social media," said Paraboni."For instance, the use of the first-person singular pronouns I and me was very prevalent, which is considered a classic sign of depression in psychology. We also observed that depressed users frequently used the heart emoji.
"This is commonly perceived as a symbol of affection and love, but perhaps psychologists have not yet classified it as such," Paraboni said.
The researchers are currently expanding the database, refining their computational techniques, and enhancing the models to determine if they can produce a tool for future use in screening potential mental health disorder sufferers and assisting the families and friends of adolescents at risk for depression and anxiety.